Track on Social Network and Media Analysis and Mining (SNMAM)
Call for Papers
Social network is a structure formed by a set (or groups of sets) of entities (e.g. people, organizations, etc.) with some patterns of social interaction. Social Network Analysis (SNA) is focused on uncovering these patterns through the use of network science and graph theory. In the last decades, online social networks (OSNs) have become the most widely used web platforms due to the variety of services they offer to their users. This explosion has led to the diversification of both content (text, images, audio and other) and the sources (e.g. newscasts, newspapers and other companies) in OSNs. The need for processing and analyzing this content as well as understanding its impact on users' lives, has allowed for the rapid development of various techniques for mining and analysis of social media data.
In this context, the Social Network and Media Analysis and Mining (SNMAM) provides a forum that brings both researchers and practitioners to discuss research trends and techniques related to the analysis and mining of social network and media data. The 5th SNMAM event will be organized as a track of SIMBig 2020 in Lima, Peru, as an interdisciplinary venue for computer scientists, computer engineers, software engineers and application developers who work on networks and web-based methods.
Therefore, SNMAM welcomes experimental and theoretical works on analysis and mining of social network and social media data along with their application to real-world problems. Young scientists and researchers from scientific centers, students and graduates, as well as industrial partners are welcome to participate.
Scope and Topics
SNMAM includes all the topics related to social network and media analysis and mining. The topics suitable for SNMAM include, but not limited to:
- Crowdsourcing of social network and media data generation and collection
- Data preparation and data modeling for social networks and social medias
- Exploratory and visual data analysis of social network and media data
- Identification and discovery of dynamics and evolution patterns of social network and media data based on data mining and machine learning approachess
- Topological and spatio-temporal aspects in social networks and social media
- Large-scale graph, search and time series algorithms on social networks
- Social network analysis and mining tasks:
- Anomaly and outlier detection
- Community discovery
- Link and node classification
- Link and node prediction
- Entity resolution
- (Social) Graph construction
- Data mining applications on social network and media data:
- Recommender systems
- Opinion and suggestion mining
- Sentiment analysis
- Misbehavior detection
- Activity prediction
- Event detection
- (Epidemic) Spreading
- Applications of social network and media in business, engineering, scientific, medical and public health domains, terrorism and/or criminology, fraud detection, politics, cyberbullying, and case studies
- Special case study: social behavior analysis due to COVID-19
- Analysis and mining of location-based social networks, urban (social) networks, multilayer social networks and others
- Social media monitoring and analysis
- Ethics, privacy and security in online social networks and social media platforms
- Tools and infrastructures for social networking platforms and web communities
- New applications and services arising from big data, social network analysis and social media.
Paper Submission Guidelines
- Papers are limited to a total of 14 (fourteen) pages, including all content and references. All submissions must be in English.
- Submissions must be in PDF, formatted with the Springer Publications format. For details on the Springer style, see here.
Easychair Submissions Website
Submissions for SNMAM 2020 here.
PROGRAM COMMITTEE
- Alexandre Donizeti Alves, Federal University of ABC, Brazil;
- Alonso Inostrosa-Psijas, Universidad Arturo Prat, Chile;
- Ankur Singh Bist, Signy Advanced Technology, India;
- Aurea Soriano Vargas, University of Campinas, Brazil;
- Brett Drury, LIAAD-INESC TEC, Portugal;
- Carlos Andrés Ferrero, Instituto Federal de Santa Catarina, Brazil;
- Conceição Rocha, INESC TEC, Portugal;
- Daniela Godoy, UNICEN University, Argentina;
- Diego Furtado Silva, Universidade Federal de São Carlos, Brazil;
- Francisco Rodrigues, University of São Paulo, Brazil;
- Geraldo Pereira Rocha Filho, University of Brasilia, Brazil;
- Hugo D. Calderon Vilca, National University of San Marcos, Peru;
- Jorge Poco, FGV EMAp, Brazil;
- Josimar Chire, University of São Paulo, Brazil;
- Luca Rossi, Queen Mary University of London, UK;
- Leandro Anghinoni, University of São Paulo, Brazil;
- Maria Lígia Chuerubim, Universidade Federal de Uberlândia, Brazil;
- Mathieu Roche, CIRAD, TETIS and University of Montpellier, France;
- Muhammad Nihal Hussain, University of Arkansas at Little Rock, USA;
- Murilo Naldi, Universidade Federal de São Carlos, Brazil;
- Katarzyna Musial-Gabrys, University of Technology Sydney, Australia;
- Kiran Kumar Bandeli, Walmart Inc, USA;
- Newton Spolaôr, Western Paraná State University, Brazil;
- Nils Murrugarra, Snap Inc, USA;
- Paola LLerena Valdivia, INRIA Saclay, France;
- Pascal Poncelet, University of Montpellier, France;
- Pedro Shiguihara Juárez, Pontifical Catholic University of Peru, Peru;
- Rafael Giusti, Federal University of Amazonas, Brazil;
- Rafael Delalibera Rodrigues, University of São Paulo, Brazil;
- Rafael Santos, National Institute for Space Research (INPE), Brazil;
- Renan de Padua, iFood, Brazil;
- Renato Fabbri, University of São Paulo, Brazil;
- Ricardo Campos, Polytechnic Institute of Tomar and LIAAD-INESC TEC, Portugal;
- Ronaldo Prati, Federal University of ABC, Brazil;
- Ryan Rossi, Adobe Research, USA;
- Sabrine Mallek, Université de Lorraine, France;
- Solange Oliveira Rezende, University of São Paulo, Brazil;
- Sultan Orazbayev, Center for International Development at Harvard Kennedy School, USA;
- Thiago de Paulo Faleiros, University of Brasilia, Brazil;
- Tiago Colliri, University of São Paulo, Brazil;
- Victor Stroele, Federal University of Juiz de Fora, Brazil;
- Willy Ugarte, Universidad Peruana de Ciencias Aplicadas, Peru;
- Zhao Liang, University of São Paulo, Brazil.
Organizers
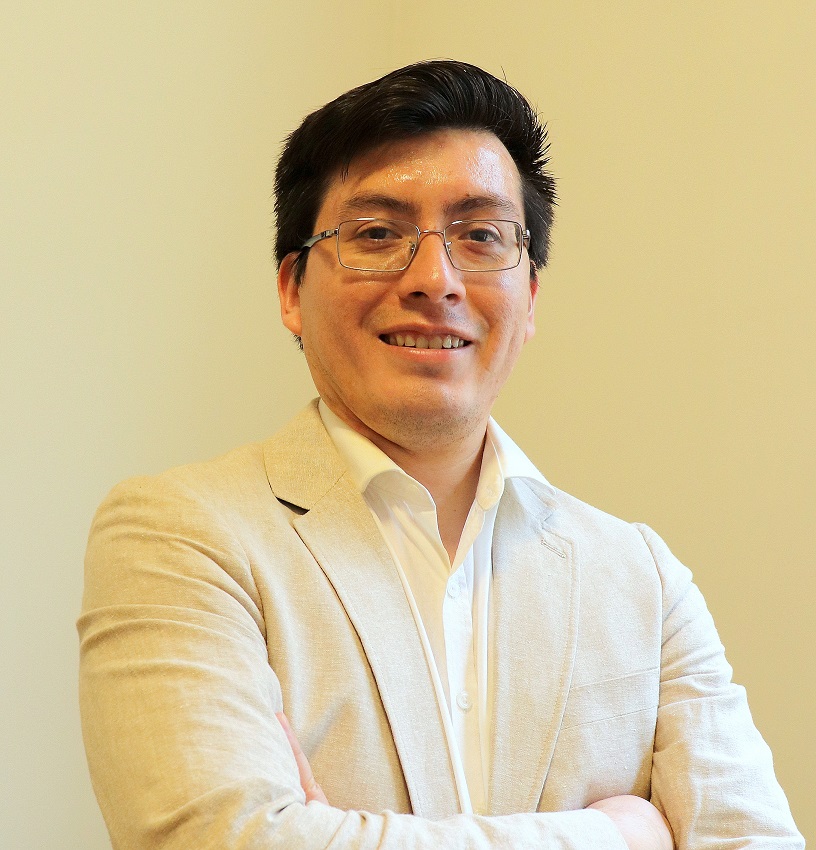
Jorge Valverde-Rebaza
PhD in Computer Science
Department of Scientific
Research, Visibilia,
BRAZIL
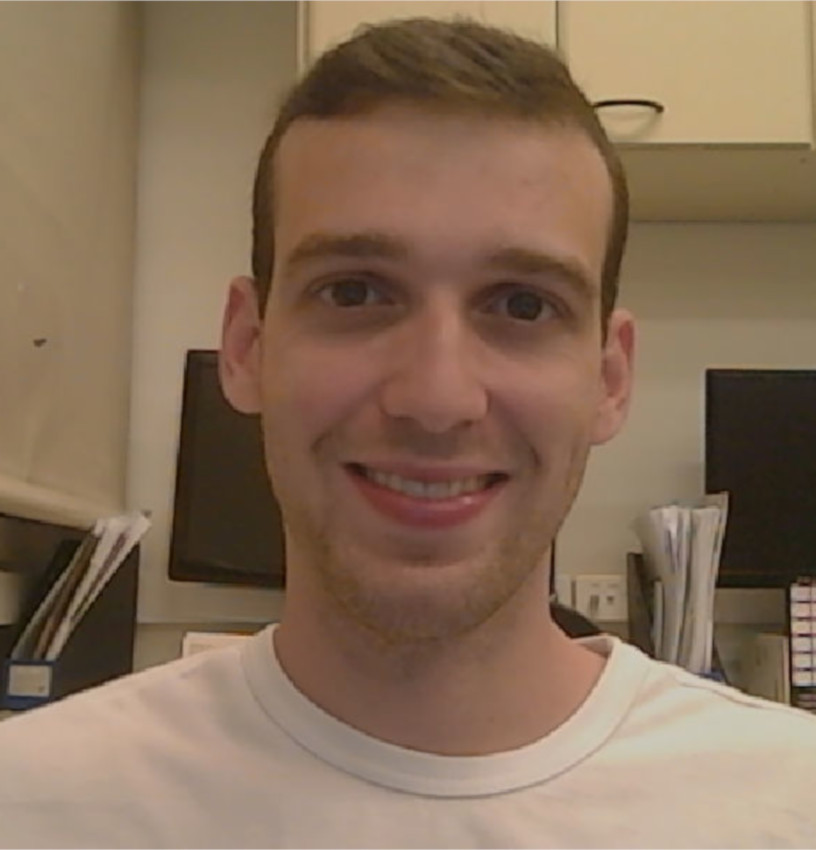
Alan Valejo
PhD in Computer Science
Department of Computing and
Mathematics (DCM), FFCLRP,
University of São Paulo (USP), BRAZIL